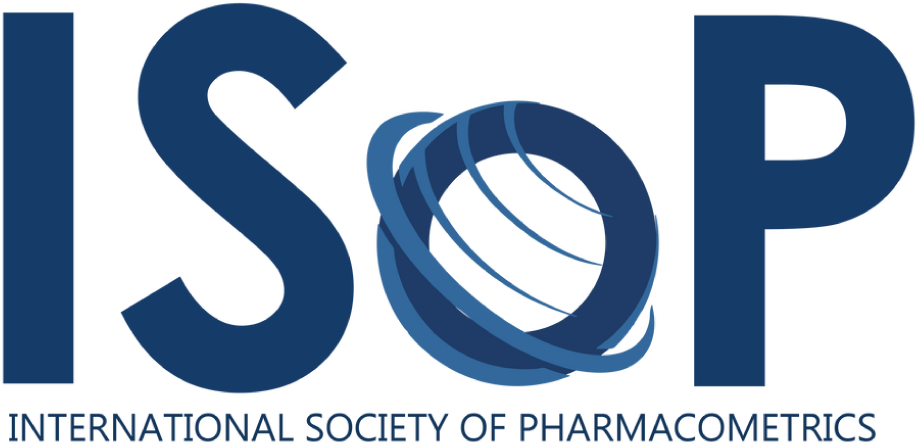
Catalog Advanced Search
-
Contains 1 Component(s) Includes a Live Web Event on 07/16/2025 at 12:00 PM (EDT)
The MIDD Webinar Series, coordinated by Yash Kapoor and Fulya Akpinar Singh, is a series of webinars focused on shaping the future of drug development and regulatory decision-making sponsored by the ISoP Education Committee. Topics range from MIDD approaches in regulatory submission to pharmacometrics topics that are at the core of model development.
The MIDD Webinar Series, coordinated by Yash Kapoor and Fulya Akpinar Singh, is a series of webinars focused on shaping the future of drug development and regulatory decision-making sponsored by the ISoP Education Committee. Topics range from MIDD approaches in regulatory submission to pharmacometrics topics that are at the core of model development.
Phyllis Chan
Pharmacometrician in Clinical Pharmacology Modeling & Simulation Group
Genentech
Phyllis is a pharmacometrician in the Clinical Pharmacology Modeling & Simulation group at Genentech in South San Francisco. Before joining Genentech in 2017, Phyllis worked at Bristol-Myers Squibb, obtained her PhD in Pharmaceutical Sciences from Auburn University and was a postdoctoral fellow at University of Southern California's Biomedical Simulations Resource (BMSR). More recently, Phyllis received an MPH from Imperial College London. She specializes in population PK, exposure-response, tumor growth inhibition-overall survival, and model-based meta-analysis.
-
You must log in to register
- Member - Free!
- More Information
-
You must log in to register
-
UPCOMING LIVE!
Great data visualization is more than just charts—it’s about effective communication. This session explores principles of design that make data clear, efficient, and beautiful, ensuring your audience grasps key insights at a glance. Learn how to craft visualizations that not only look good but also enhance understanding and tell a compelling story with your data.
Great data visualization is more than just charts—it’s about effective communication. This session explores principles of design that make data clear, efficient, and beautiful, ensuring your audience grasps key insights at a glance. Learn how to craft visualizations that not only look good but also enhance understanding and tell a compelling story with your data.
Dr. Cédric Scherer
Dr. Cédric Scherer (he/him) is a computational ecologist turned independent data visualization professional. Combining research expertise with a passion for data and design, he helps organizations, research teams, and businesses communicate insights through engaging visualizations, reports, and presentations. Specializing in bespoke, code-driven designs—from clear and effective to artistic and unconventional—Cédric has created visualizations across various disciplines and regularly teaches data visualization principles, R, and ggplot2.
-
You must log in to register
- Member - Free!
- More Information
-
You must log in to register
-
Contains 1 Component(s) Includes a Live Web Event on 06/18/2025 at 12:00 PM (EDT)
The MIDD Webinar Series, coordinated by Yash Kapoor and Fulya Akpinar Singh, is a series of webinars focused on shaping the future of drug development and regulatory decision-making sponsored by the ISoP Education Committee. Topics range from MIDD approaches in regulatory submission to pharmacometrics topics that are at the core of model development.
The MIDD Webinar Series, coordinated by Yash Kapoor and Fulya Akpinar Singh, is a series of webinars focused on shaping the future of drug development and regulatory decision-making sponsored by the ISoP Education Committee. Topics range from MIDD approaches in regulatory submission to pharmacometrics topics that are at the core of model development.
Rong Chen
Research Scientist
Certara
Rong is a research scientist at Certara USA Inc’s Data Sciences Division Phoenix NLME group. His responsibility involves maintaining, improving, and developing the existing and new computational and statistical algorithms for Phoenix NLME software. Rong got his nuclear physics PhD at Arizona State University in 2020. The PhD focused on large-scale quantum Monte Carlo simulations. Prior to join Certara in 2023, Rong was a postdoctoral research fellow at Children’s Hospital Los Angeles where he developed high performance parametric and nonparametric algorithms for population pharmacokinetics/pharmacodynamics (PK/PD) modeling.
-
You must log in to register
- Member - Free!
- More Information
-
You must log in to register
-
Contains 1 Component(s) Includes a Live Web Event on 05/21/2025 at 12:00 PM (EDT)
The MIDD Webinar Series, coordinated by Yash Kapoor and Fulya Akpinar Singh, is a series of webinars focused on shaping the future of drug development and regulatory decision-making sponsored by the ISoP Education Committee. Topics range from MIDD approaches in regulatory submission to pharmacometrics topics that are at the core of model development.
The MIDD Webinar Series, coordinated by Yash Kapoor and Fulya Akpinar Singh, is a series of webinars focused on shaping the future of drug development and regulatory decision-making sponsored by the ISoP Education Committee. Topics range from MIDD approaches in regulatory submission to pharmacometrics topics that are at the core of model development.
Yuezhe Li
Research Scientist II
Metrum Research Group
Yuezhe Li is a Research Scientist II at Metrum Research Group. Since joining Metrum Research Group in 2021, her work has focused on developing quantitative systems pharmacology (QSP) models to support preclinical and clinical development of antibody drug conjugates (ADCs), bispecific T cell engagers, and gene therapies. Yuezhe earned her Ph.D. in Biomedical Science from the University of Connecticut. Other fields of experiences include systems biology, machine learning, and mathematical modeling.
-
You must log in to register
- Member - Free!
- More Information
-
You must log in to register
-
On-Demand
An important skill for quantitative scientists within the pharmaceutical industry is the need effectively communicate complex technical information. This is especially challenging when the audience includes high-level stakeholders such as senior managers and regulatory representatives who may not have a deep technical understanding but must rely on modeling and simulation analyses to drive decision making. In this webinar, important points to consider will be discussed that can help attendees develop and deliver successful presentations that are tailored to meet the needs of the target audience and their objectives.
An important skill for quantitative scientists within the pharmaceutical industry is the need effectively communicate complex technical information. This is especially challenging when the audience includes high-level stakeholders such as senior managers and regulatory representatives who may not have a deep technical understanding but must rely on modeling and simulation analyses to drive decision making. In this webinar, important points to consider will be discussed that can help attendees develop and deliver successful presentations that are tailored to meet the needs of the target audience and their objectives.
Ginny Schmith
Virginia (Ginny) Schmith has 35+ years of experience in clinical pharmacology and pharmacometrics providing strategy across all phases of drug development and numerous therapeutic areas: anesthesia, cardiovascular, pulmonary, gastrointestinal, central nervous system, dermatology, cancer, inflammation, antibiotics, antivirals, and rare diseases, and has actively contributed to the approval of 15+ drugs. She worked for GSK (and predecessor companies) for 26 years and then went into consulting at Nuventra Pharma Sciences for almost 7 years. Currently, she continues to consult with small companies within her own company (Schmith PK/PD Consulting LLC) and as part of the Syntegrity Quantitative Clinical Pharmacology Collaborative.
Ginny’s focus is on the strategy for using quantitative clinical pharmacology approaches to answer drug development questions, even when there is incomplete data, and communicating (written and orally) this information to regulatory agencies and management within small biotechnology companies.
Dr. Schmith has been an Adjunct Professor at UNC-CH Eshelman School of Pharmacy since 1989 and recently become a Courtesy Clinical Professor at the University of Florida School of Pharmacy. Ginny has published 50 peer-reviewed articles and reviews in international scientific journals in addition to over 80 published abstracts related to clinical pharmacology and pharmacometrics; she has authored 6 patents; and is an active member of the American Society of Clinical Pharmacology (since 1989), the International Society of Pharmacometrics, and a fellow of the American College of Clinical Pharmacology.
-
You must log in to register
- Member - Free!
- More Information
-
You must log in to register
-
Contains 1 Component(s) Recorded On: 04/16/2025
The MIDD Webinar Series, coordinated by Yash Kapoor and Fulya Akpinar Singh, is a series of webinars focused on shaping the future of drug development and regulatory decision-making sponsored by the ISoP Education Committee. Topics range from MIDD approaches in regulatory submission to pharmacometrics topics that are at the core of model development.
The MIDD Webinar Series, coordinated by Yash Kapoor and Fulya Akpinar Singh, is a series of webinars focused on shaping the future of drug development and regulatory decision-making sponsored by the ISoP Education Committee. Topics range from MIDD approaches in regulatory submission to pharmacometrics topics that are at the core of model development.
Nadia Noormohamed
Clinical Pharmacologist
GSK
Nadia is a clinical pharmacologist currently working in the CPMS group at GSK within Vaccines and Infectious Disease. For the past 3 years, she has worked on MIDD approaches in the HBV space, with a focus on PKPD modeling. She has also contributed to internal and external initiatives in the areas of organ impairment and QT prolongation. Nadia had initially trained as a pharmacist and graduated with a PharmD from Massachusetts College of Pharmacy. Research experience while in pharmacy school motivated her to pursue a postdoc in pharmacometrics as part of a combined program with Merck and Rutgers University. After her postdoc and prior to joining GSK in her current role, she continued with Merck in the Pharmacometrics group and gained hands-on experience in various therapeutic areas. Outside of work, Nadia enjoys reading, powerlifting, and perusing different neighborhoods in Philadelphia.
-
You must log in to register
- Member - Free!
- More Information
-
You must log in to register
-
Contains 1 Component(s) Recorded On: 03/31/2025
We consider nonlinear mixed effects models including high-dimensional covariates to model individual parameters variability. The objective is to identify relevant covariates and estimate model parameters. We combine a penalized LASSO-type estimator with an eBIC model choice criterion to select the covariates of interest. Then we estimate the parameters by maximum likelihood in the reduced model. We calculate the LASSO-type penalized estimator by a weighted proximal gradient descent algorithm with an Adagrad-type adaptive step. This choice allows us in particular to consider models that do not necessarily belong to the curved exponential family. We illustrate the performance of the method in a nonlinear mixed-effects logistic growth model.
We consider nonlinear mixed effects models including high-dimensional covariates to model individual parameters variability. The objective is to identify relevant covariates and estimate model parameters. We combine a penalized LASSO-type estimator with an eBIC model choice criterion to select the covariates of interest. Then we estimate the parameters by maximum likelihood in the reduced model. We calculate the LASSO-type penalized estimator by a weighted proximal gradient descent algorithm with an Adagrad-type adaptive step. This choice allows us in particular to consider models that do not necessarily belong to the curved exponential family. We illustrate the performance of the method in a nonlinear mixed-effects logistic growth model.
Estelle Kuhn
Researcher in Statistics
INRAE - University Paris Saclay
Estelle Kuhn, Senior Researcher in Statistics, at INRAE-University Paris Saclay I am senior researcher in statistics at INRAE-University Paris Saclay in the Dynenvie team at the Applied Mathematics and Computer Science from Genome to Environment lab (MaIAGE). My current research topics concern inference and variable selection in high-dimension setting in latent variable models such as mixed and joint models. I am also interested in hybrid models combining mechanistic and statistical models. I develop stochastic optimization algorithms such as expectation maximization and gradient descent adapted to different contexts. I apply these methodologies in strong collaboration with colleagues at interfaces, particularly in plant breeding and animal genetics.
-
You must log in to register
- Non-member - Free!
- Member - Free!
- Guest User - Free!
- More Information
-
You must log in to register
-
Contains 1 Component(s) Recorded On: 03/26/2025
The MIDD Webinar Series, coordinated by Yash Kapoor and Fulya Akpinar Singh, is a series of webinars focused on shaping the future of drug development and regulatory decision-making sponsored by the ISoP Education Committee. Topics range from MIDD approaches in regulatory submission to pharmacometrics topics that are at the core of model development.
The MIDD Webinar Series, coordinated by Yash Kapoor and Fulya Akpinar Singh, is a series of webinars focused on shaping the future of drug development and regulatory decision-making sponsored by the ISoP Education Committee. Topics range from MIDD approaches in regulatory submission to pharmacometrics topics that are at the core of model development.
Mayu Osawa
Director in Pharmacometrics
Bristol Myers Squibb
Mayu Osawa has over a decade of experience in the pharmaceutical industry at Bristol Myers Squibb, where she serves as a Director in Pharmacometrics. She has extensive experience in both early and late development programs, leading modeling work across therapeutic areas including oncology, immunology, and hematology to support drug development decisions, dose selection, and health authority submissions. She obtained her Ph.D. in biostatistics from Kitasato University.
Géraldine Cellière
VP Applications Clinical Pharmacology & Pharmacometrics Solutions
Simulations Plus
Dr Géraldine Cellière is a multidisciplinary scientist with a background in statistics and modeling. She holds an engineering degree from Ecole Polytechnique (Paris, France) and a Master in computational biology from ETH Zürich (Switzerland). After an experience as assistant project manager at SoBios, she did a PhD in systems biology and multi-scale modeling at INRIA Paris and received her doctoral degree in 2016. Géraldine then joined Lixoft (now part of Simulations Plus) as VP Application. There, she leads the team in charge of all pre- and post-sales activities (demonstrations, trainings, technical support), research activities (improvement and development of new methods, application to case studies, publications), as well as the MonolixSuite development (product specification and testing).
-
You must log in to register
- Member - Free!
- More Information
-
You must log in to register
-
On-Demand
In November 2024, the ICH Assembly endorsed the draft guideline entitled “M15 General Principles for Model-Informed Drug Development” and agreed to make the guideline available for public comment. This marks a major milestone for model-informed drug development (MIDD) and its maturity as a globally accepted standard for evidence generation. The draft guideline is the product of the multidisciplinary Expert Working Group (EWG) of the ICH. It establishes a harmonized assessment framework (including associated terminology) for MIDD evidence and provides an assessment table as a tool for communication. In this session, members of the ICH M15 EWG from regulatory agencies and industry will provide an overview of the draft guideline. The presentations will cover key contents of the M15 guideline, relevant perspectives, and examples.
In November 2024, the ICH Assembly endorsed the draft guideline entitled “M15 General Principles for Model-Informed Drug Development” and agreed to make the guideline available for public comment. This marks a major milestone for model-informed drug development (MIDD) and its maturity as a globally accepted standard for evidence generation. The draft guideline is the product of the multidisciplinary Expert Working Group (EWG) of the ICH. It establishes a harmonized assessment framework (including associated terminology) for MIDD evidence and provides an assessment table as a tool for communication. In this session, members of the ICH M15 EWG from regulatory agencies and industry will provide an overview of the draft guideline. The presentations will cover key contents of the M15 guideline, relevant perspectives, and examples.
Scott Marshall
VP, Clinical Pharmacology
GSK
As CPMS head for Asia, Scott is in a key role in the rapid expansion of the GSK footprint in North/East Asia, given its ongoing commitment to the discovery and development of new therapies that deliver improved outcomes for patients globally.
Scott gained his Ph.D. in PK/PD modeling and simulation from the Department of Medicine and Therapeutics, University of Glasgow. He has since had a wide range of leadership roles associated with the evolution of Model Informed Drug Development (MIDD) practice, tool, methods and process development over his 25 years at Pfizer. He worked as a Clinical Pharmacologist (CP) and/or Pharmacometrician (PMx) across cardiovascular, sexual health, genital urinary and pain disease areas. He has published over 35 papers on the application of MIDD in development of a range of therapeutics as well as notable good practice and interdisciplinary applications integrating MIDD with statistical and pharmacoeconomic approaches.
He was lead author on EFPIA MID3 good practice white paper and co-led associated regulatory interactions. Scott is currently the Rapporteur for the ICH MIDD Discussion group with a one-year remit to align on ICH MIDD guideline development strategy. Notably this group of global regulatory and industry experts have now aligned on a path forward for ICH MIDD related guideline. A key output is the alignment on the scope and value of a MIDD general principles guideline which is being development M15 IWG/EWG. A road map covering other potential ICH guidelines and concept paper are currently available.
Scott is a member of GSK CPMS leadership team. He has a remit which extends to supporting the integration of MIDD approaches with other quantitative disciplines and evolving the Clinical pharmacology role to help deliver on GSK’s mission with respect to efficient global drug development and simultaneous submission.
Sarem Sarem
Senior Assessor
Biostat and Pharmacometrics Unit, Office of Science, Pharmaceutical Drug Directorate, Health Canada
Dr. Sarem Sarem is Co-Chair of the pharmacometrics working group in Health Products and Food Branch and a senior pharmacometrics assessor at the pharmaceutical drugs directorate in Health Canada, where Sarem provides internal consultation and expert review for advanced modeling and simulation studies supporting drug claims and clinical trial designs. Sarem is a topic leader in the EWG for ICH MIDD Guidance. Sarem has a PhD in pharmacometrics from the University of Montreal and work expertise as a pharmacist and in the pharmaceutical industry.
Malidi Ahamadi
US Head of Modeling and Simulation
Sanofi
Dr. Malidi Ahamadi is the Head of Modeling and Simulation Pharmacokinetics, Dynamics and Metabolism at Sanofi, US. Over the past 15 years, Malidi has an extensive academic and industrial experiences in applying modeling and simulation strategies within different scientific fields including the application of MIDD strategy in drug development. Malidi is the deputy topic leader representing the Biotechnology Innovation Organization (BIO: https://www.bio.org/) in the EWG for ICH MIDD Guidance.
Jenny Chien
Associate VP Global PK/PD & Pharmacometrics
Eli Lilly and Company
Dr. Chien is an associate VP and a scientific lead in the Global PK/PD and Pharmacometrics Department at Eli Lilly and Company. She received her PhD degree in Pharmaceutical Sciences from the University of Washington, Seattle in 1997. She is recognized for her expertise in applying MIDD strategy in trial design optimization and dose-finding from Drug Discovery and Development. She currently serves as the PhRMA Topic Leader on ICH M15.
Mark Peterson
Senior Director, Clinical & Quantitative Pharmacology
Vertex Pharmaceuticals
Dr. Mark C. Peterson is a Senior Director in the Clinical & Quantitative Pharmacology department at Vertex Pharmaceuticals. Mark received a Bachelor of Sciences in Pharmacy (1992) and a Doctorate in Pharmaceutical Sciences (2000) from the University of Connecticut. Over his 24-year industry career, he has served as a disease area lead within companies and a session and meeting organizer, invited speaker, and author on pharmacometrics related topics within the scientific community. Mark has applied a broad range of modeling and simulation-based methods from Bayesian to quantitative systems pharmacology (QSP) to address pre-clinical through registration stage questions/challenges across numerous modalities and disease areas. For the ICH Model Informed Drug Development MIDD Guidance (M15), Mark serves as the Biotechnology Innovation Organization (BIO) Topic Leader.
-
You must log in to register
- Non-member - Free!
- Member - Free!
- Guest User - Free!
- More Information
-
You must log in to register
-
Contains 1 Component(s) Recorded On: 02/18/2025
The MIDD Webinar Series, coordinated by Yash Kapoor and Fulya Akpinar Singh, is a series of webinars focused on shaping the future of drug development and regulatory decision-making sponsored by the ISoP Education Committee. Topics range from MIDD approaches in regulatory submission to pharmacometrics topics that are at the core of model development.
The MIDD Webinar Series, coordinated by Yash Kapoor and Fulya Akpinar Singh, is a series of webinars focused on shaping the future of drug development and regulatory decision-making sponsored by the ISoP Education Committee. Topics range from MIDD approaches in regulatory submission to pharmacometrics topics that are at the core of model development.
Ana Victoria Ponce-Bobadilla
Principal Pharmacometrician
AbbVie
Ana Victoria Ponce-Bobadilla is a Principal Pharmacometrician at AbbVie, focusing on MIDD in immunology and neuroscience. In her role, she develops PK-PD modeling approaches and integrates state-of-the-art machine learning frameworks to support internal projects and regulatory submissions. She holds a Ph.D. in Applied Mathematics from Heidelberg University, Germany, a Master's in Complex System Science from École Polytechnique, France, and the University of Warwick, UK, and a Bachelor in Mathematics from UNAM, Mexico. She has a strong interest in the integration of mechanistic modeling with machine learning, aiming to drive significant advancements in clinical pharmacology.
-
You must log in to register
- Member - Free!
- More Information
-
You must log in to register